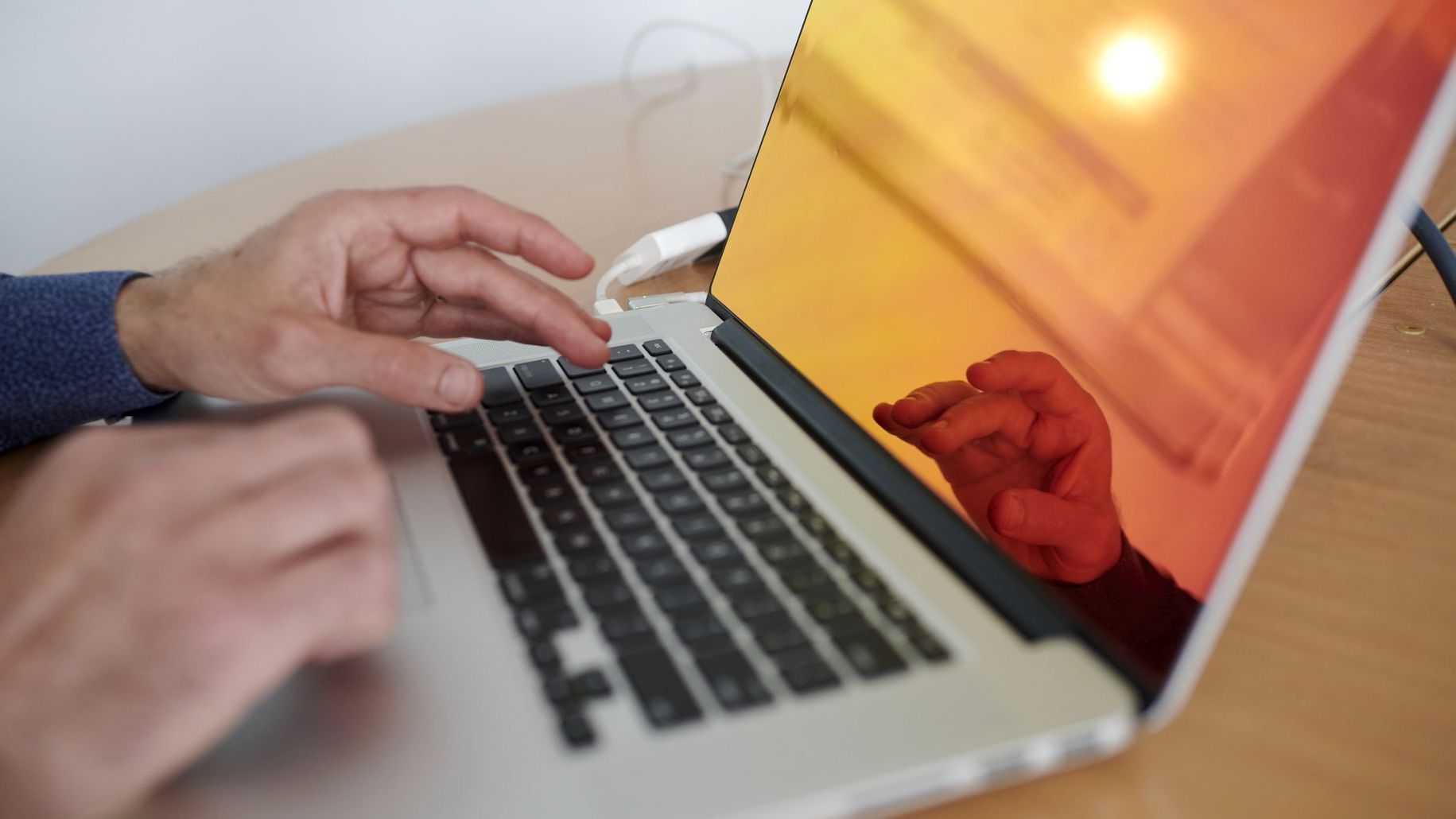
What defines responsible AI?
Artificial intelligence is a set of software programmes and methods which is neither responsible nor irresponsible in itself; it is the organisation, processes and human beings behind AI that must be responsible. And they must be responsible not only in their intent, but above all in their actions and effects. We are not making judgements about a science, but about the people who create or oversee the algorithms. In their interactions with humans, algorithms can behave in a malicious, disloyal, misleading or biased manner. The interactions of AI algorithms with humans are overseen by a type of ethical quality standard. If this interaction conforms to the defined standard, we can consider it to be responsible. Responsibility is ethical and legal but also environmental.
Is AI increasingly irresponsible?
The technological frenzy associated with the progress made in AI, the frantic pursuit of innovation, and the increasingly massive volumes of data, all increase the temptations to act irresponsibly. Where irresponsibility exists, it is generally because there is an overwhelming quest for performance which ignores responsibility, be it consciously or unconsciously. For example, certain home delivery companies are tempted to use their deliverers’ personal data, such as the number days of sick leave taken, to assess their performance. If this temptation is not controlled – by the organisations themselves and by the State – it becomes irresponsible. Therefore, it is not the algorithm that is irresponsible, but the manner in which it is used in the organisation of labour, and specifically for scheduling or pay. In France, we have a fairly effective legal control apparatus; aggressive or biased commercial practices are punished by the Directorate-General for Competition, Consumer Affairs and Fraud Control, for example.
However, an institution such as INRIA is confronted with a scientific question: how can we prove that an algorithm is biased? Several of our project teams, such as Privatics, Dyonisos, Wide and Magnet are working on this issue.
Who are the stakeholders concerned by AI responsibility?
There are three stakeholders: the public sector, the private sector and civil society. In the public sector, a number of initiatives highlight the question of responsibility. Task forces such as Etalab, which coordinates the design and implementation of government strategy in the field of data and testifies to the State’s self-regulation, come to mind. In the private sector, we have two different cases: virtuous companies or organisations which incorporate this responsibility into their values, on the grounds of ethics and/or reputation; and then there are those companies that see no benefit in addressing the issue of responsibility, which wait to be punished before changing their behaviour, or which have been punished and have not yet sufficiently modified their practices. This applies to companies in a dominant position, for example. The “Facebook Papers” are a flagrant revelation of the absence of self-regulation in certain firms.
The public sector, while far from perfect, is bound by transparency-related constraints – this is a constitutional requirement. Well-intentioned private operators adopt the tools and processes they need to become more responsible. For such players, we must create a range of support and training schemes to help them continue along this path toward virtuous practices. We must also improve the dissemination of scientific culture, particularly in AI, throughout the industrial fabric, and work with professional federations to build solid training programmes.
Wilfully irresponsible or negligent private operators do not respond positively to a co-construction-based approach. You would have to be irrational or naive to believe that such companies would naturally follow the path to regulation via a collaborative approach. Such operators need to be targeted by a supervisory framework.
In the midst of all these players, INRIA is a neutral power which can provide advice and explanations corroborated by long-term research – a legitimate need in order to make sense of the issues and provide the hindsight that other players sometimes lack. INRIA can also contribute to the creation of a shared digital asset, in the move towards more explainable, controllable and ultimately more responsible AI in decision-making.
Is there a European policy on supervision and regulation?
France is at the forefront of these matters. Alongside Germany and the Netherlands, for example, France is spearheading the development of the DSA and DMA, two European rules aimed at regulating the digital environment. Regulation and supervision will ultimately be on a European level, no doubt with a handful of countries leading the way. The regulatory bodies of Member States are entrusted with specific aspects of supervision according to local priorities and expertise.
Nevertheless, it is sometimes difficult to enable the coexistence of different perceptions of discrimination or detrimental conduct, for example, on major social networks, which are merely a reflection of ideological sensitivities or regional policies. Content-moderation algorithms on large platforms, which are highly sensitive to language, produce very different results from one country to another. This calls for extensive collaboration between countries, on economic issues, but also in relation to cultural and legislative questions.