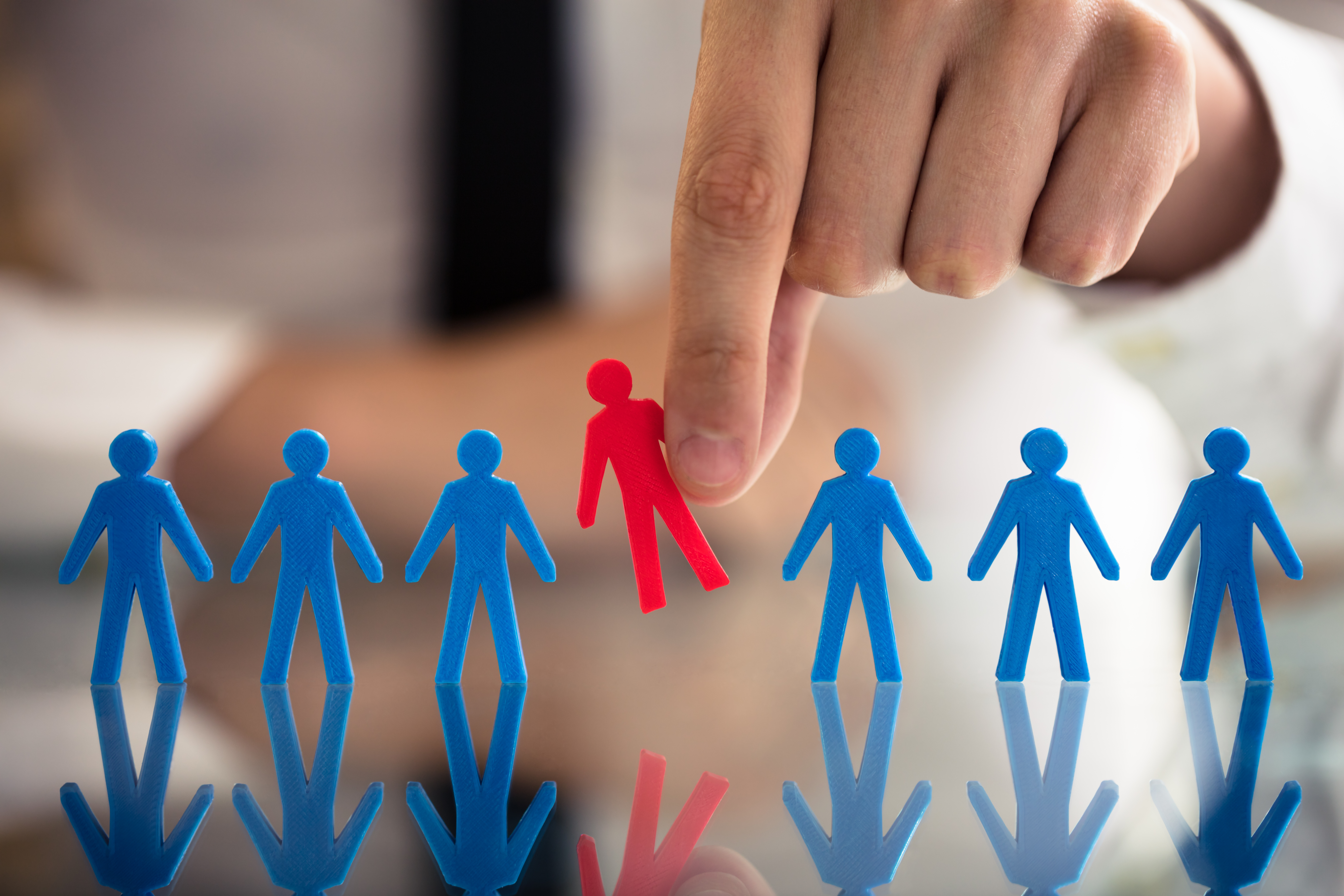
Machine learning is an integral, inseparable part of artificial intelligence (AI) and has developed considerably over the last twenty years. The purpose is to train a model to respond to a problem by presenting it with a multitude of examples that represent the task to be performed. This technology is having an increasingly significant impact on society, but it is sometimes criticised for its lack of equity. On several occasions, learning algorithms have been accused of discriminating against certain categories of the population (based on gender, social backgrounds, etc.).
"Just as in the early 20th century philosophers examined changes in society as a result of which institutions began to make important decisions, such as legal and sentencing decisions, instead of individuals, it is essentialthat we analyse these algorithms from the perspective of their societal impact," says Gaurav Maheshwari, who worked on his thesis at Inria as part of the Magnet project team, finishing in 2024. The aim of his research was to investigate and propose ways of measuring and mitigating the negative impact of societal biases. These are inherent in the data used to train the algorithms, reflecting the inequalities specific to the societies in which the data is collected.
Societal biases likely to increase discrimination
How can we define these biases? Gaurav Maheshwari quotes Kate Crawford, a leading theorist on AI’s social and political implications, who has identified two major types of bias associated with machine learning: allocation bias and representation bias. The first occurs when population groups are unfairly allocated or denied opportunities and resources as a result of algorithmic intervention. The second arises when algorithm systems perpetuate and amplify stereotypes associated with certain social groups.
Gaurav Maheshwari received considerable support for his research: his thesis was part of the ANR SLANT project (Spin and Bias in Language Analyzed in News and Text),which came to an end in 2024. As well as Inria, this project involved the Toulouse Research Institute for Computer Science (IRIT) and the University of Luxembourg.
"The aim of the ANR project was to characterise political bias in the media, but we agreed to continue working on allocation bias. Researchers in Toulouse and Luxembourg worked on representation bias," says Mikaela Keller, associate professor at the University of Lille, who co-supervised the PhD with two researchers from the Magnet team, Aurélien Bellet and Pascal Denis. "The two types of bias are linked: representation bias very probably affects resource allocation, and by correcting allocation bias, we can assume that we are implicitly correcting certain representation biases", she says.
Open-source software to correct bias
Surprisingly, even when an algorithm is based on anonymised data, bias can emerge due to elements associated with stereotypes. A typical example is automated CV screening based on existing profiles.
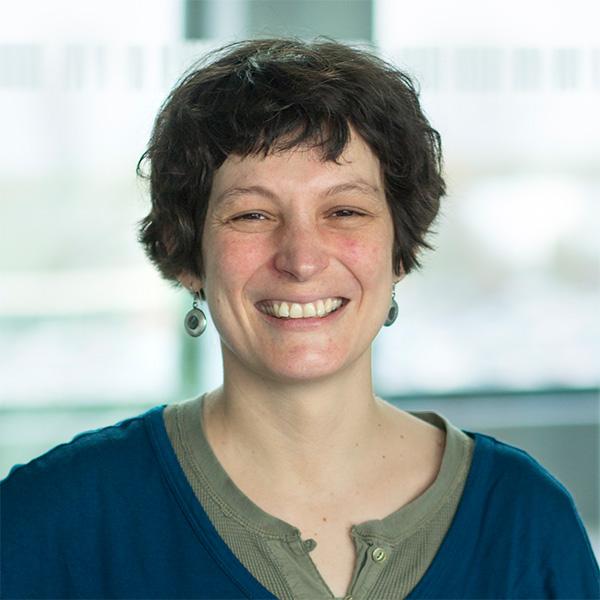
Verbatim
If the CVs are predominantly male, as in the IT sector, then the algorithm is likely to reject female CVs, even if gender is not explicitly mentioned. The different ways in which men and women are socialised lead to biases that make it possible to identify a person's gender. For example, the frequency with which certain words are used.
Associate professor at the University of Lille
So how can we effectively address this phenomenon? "In response, we are proposing a series of algorithms designed to measure and mitigate the harm associated with inequitable resource allocation throughout the machine learning process," says Gaurav Maheshwari. With Michaël Perrot, he has developed software called FairGrad (Fairness Aware Gradient Descent), which dynamically increases the importance of the data of disadvantaged people, while reducing the impact of advantaged people.
This learning method has been released as an open-source program, making it easy to adapt to existing systems. "Thanks to experiments carried out on over ten datasets and six basic models, we have demonstrated that FairGrad is an efficient processing method, offering wide applicability with limited computational overhead", says Gaurav Maheshwari.
Taking intersectionality into account
But the researcher and his teammates didn't stop there. They then examined FairGrad from the angle of "intersectionality". In other words, they included multiple factors for discrimination simultaneously.
Verbatim
Recent studies have shown that even when equity is established between two groups for each individual criterion relating to discrimination, significant inequity can still exist at the level of intersections - when a person belongs to several groups (for example African-American women).
Ex-PhD student from the Magnet project team.
This is an unprecedented approach, as Mikaela Keller says: "working on intersectionality is one of the innovations proposed by Gaurav. Until now, there has been little research into such cases, which combine several factors for discrimination.” As a result, the team's researchers came to the following conclusion: several approaches that are supposed to promote equity actually harm the groups concerned!
At the crossroads of equity and privacy
Faced with this problem, Gaurav Maheshwari and his team have come up with a solution: a new mechanism for generating data for people at the intersection of several categories, by combining data from related groups. For example, for older African-American women, the data is based on three separate categories: older African-American people, older women and African-American women. "We show that this approach not only produces new, realistic examples, but also improves performance for the most disadvantaged groups", says the researcher.
Another research theme studied by Gaurav Maheshwari and his team is respect for data anonymity. “It's one of Magnet's major themes, so the idea came quite naturally," says Mikaela Keller. “We developed FEDERATE, a method which involves trying to 'debias' an algorithm, while preserving the anonymity of the data used. These two objectives previously seemed to be in conflict, but Gaurav has proposed a new algorithm with a certain amount of success." The initiative is very promising in the eyes of the researcher: "the work is still in its initial phase, but it advances the state of the art and represents another step towards building fairness in AI."
Find out more
- Society is biased, and that biases AIs (in French), The Conversation, 4/11/2024.
- AI decentralized: how to ensure more fairness and privacy? Inria, 02/10/2023.
- Algorithms: discrimination, sexism and racism, what you need to know (in French), Franceinfo, 21/6/2023.
- Algorithmic discrimination | 2 minutes of AI (video, in French), Sorbonne Université, 10/11/2021.
- Representativeness bias in artificial intelligence (in French), Binaire (Le Monde computer science blog), 31/8/2021.
For experts
- FairGrad : Fairness Aware Gradient Descent, par Gaurav Mahesharwari, Michaël Perrot, Transactions on Machine Learning Research, 8/2023.