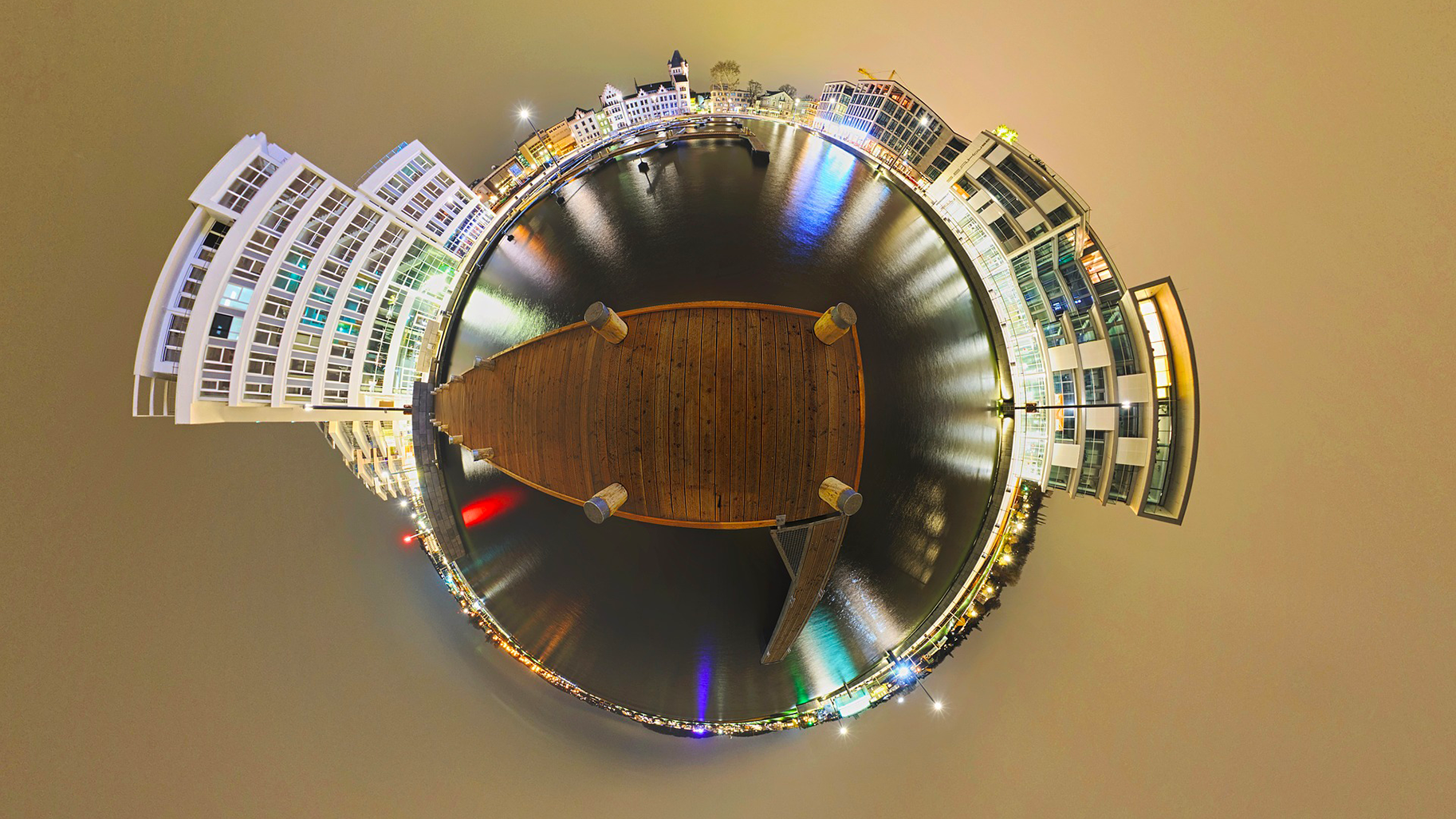
A single click to smooth out a few wrinkles, another to slim the figure, a third to add a little colour… The job’s done! In just a few years, artificial intelligence (AI) has become an integral part of image processing with astonishing results. But the famous neural network algorithms behind this success are confronted with a problem: they struggle to integrate the stunning dimension of the new 3D images produced by omnidirectional cameras or plenoptic sensors. These so-called "light field" sensors no longer simply transcribe the image in its 2D plane. They incorporate every dimension of the scene, including volume, allowing you to choose one focus point among several thousands, modify the depth of field or even change the framing.
The artificial intelligence chair held by Christine Guillemot aims to develop neural networks able to work on this scale. It follows on from a project CLIM (Computational Light Field IMaging) on these new imaging methods chosen by the European Research Council (ERC) in 2016.
The size of the data impacts the algorithms
“We had already started to work on deep learning during the ERC project. These methods work well but we can’t immediately transpose them to omnidirectional or plenoptic imaging. Firstly, because the size of the data also impacts the size of the algorithms used for learning. The neural networks already have to incorporate millions of parameters in 2D. This can cause problems, for example, for storage on mobile devices. Now, with the new imaging methods, the number of criteria to be taken into account explodes. These new images also have specific properties that must be taken into account. For example, the omnidirectional image is spherical. We must therefore also integrate this into our models.”
The new research will first consist in articulating learning and dimensionality reduction. “We suppose that the data can be represented by smaller data in subspaces, which are specific domains usually defined by dictionaries or that will be learned by neural networks.” Here, the research will use a whole range of methods that are traditionally used in signal processing and compression such as parsimonious modelling, low-rank approximation and graph-based models. “Plenoptic data can be seen, for example, as multi-view captures. The graphs will help us connect the correlated points. Thanks to the depth information of the scene, we know that a pixel with an xy position in one view is correlated with an x'y' pixel in another. But the two pixels actually correspond to the same 3D point in space. This dependency information can be represented by a line connecting two points of a graph to allow the neural network to then take it into account.”
Inverse problems
Verbatim
An inverse problem is a poorly posed problem because there is no single solution given the input observations.
The topic is also closely linked to the resolution of inverse problems as they are classically encountered in signal reconstruction: denoising, deblurring, super-resolution, compressed sampling etc. “An inverse problem is a poorly posed problem because there is no single solution given the input observations. These can be blurred or disrupted by noise if the sensor is of poor quality, if there is optical aberration or movement during capture. Depending on the device used, different observations can therefore be made from which the imaging model must trace back to the data of the real scene.”
For the time being, "in the literature, we learn a neural network for each type of inverse problem. One for denoising, one for super-resolution, etc. We would like to obtain a single network that can be used for all these cases. It would be a question of representing an a priori on the image, on the data we are aiming to reconstruct, and being able to use this deep a priori in traditional signal processing algorithms to combine the optimisation methods.”
There is an additional difficulty, however: “to function well, neural networks must be supplied with large amounts of learning data. Millions of examples. But plenoptic and omnidirectional technologies are recent. We don’t have that much learning data yet. We therefore risk having parameters that stick too closely to a small number of images. This is known as over-interpretation or overfitting.”
Plenoptic microscopes
The Chair will last four years. In addition to Christine Guillemot’s work, it will finance, via the French National Research Agency, a young researcher, a post-doctoral researcher, a PhD student and an engineer. There will also be a PhD student funded by Inria and two students carrying out Cifre PhDs in two partner companies operating in the image sector.
This work concerns many fields of application, including driverless cars. “Vehicle navigation is based on computer vision. The neural network is used to interpret the content in the scene. With plenoptic images, we can collect much more in-depth information than in 2D.”
In a completely different vein, "in microscopy, the 3D information will allow us to use super resolution to choose the best focal plane in the optical axis. At Stanford University, plenoptic microscopes are already at the prototype stage.”