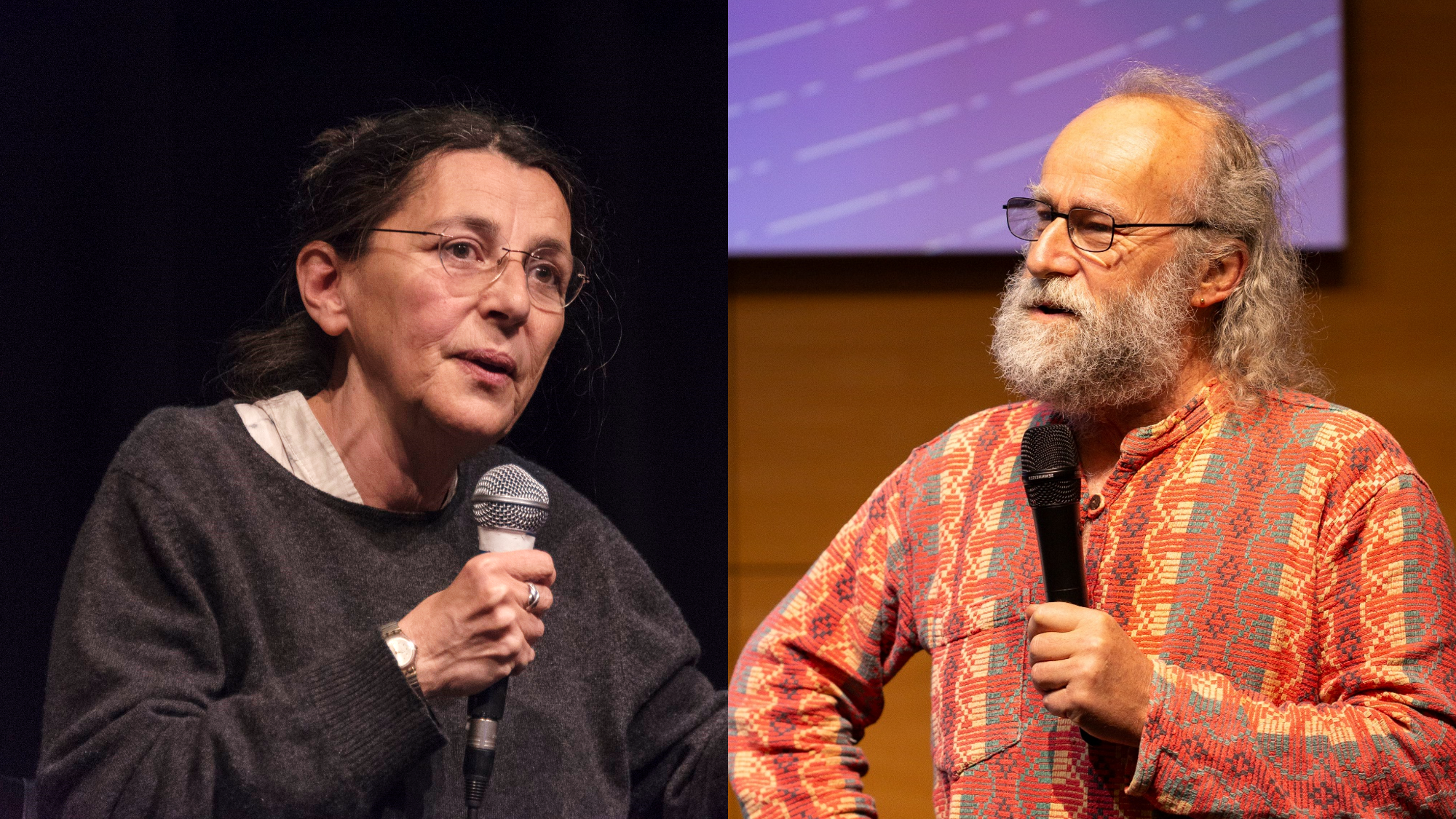
You’re both retiring just when AI seems to have settled into our day-to-day lives once and for all. What’s your assessment of the evolution of your field of research over the past few decades?
Michèle Sebag — We started in the early 1990s, at a time when AI was frowned upon. At the time, AI was a field in which you thrived if you were into “hard” sciences such as computer science and mathematics, but also economics, cognitive science or philosophy. This was unnerving both for hard scientists, who didn’t take AI researchers seriously, and for philosophers, who thought the scientists were too light-hearted about it. For multidisciplinary researchers, it was a classic case of “you cannot win”!
Marc Schoenauer — AI history has featured more than one dark period, known as “AI winters”: in the late 1970s and the early 1990s.
Each time, AI held lots of promise but produced few results. Now it’s more like summer! What has changed is that empirical achievements in machine learning, the most obvious aspect of AI, are now undeniable, even for the most sceptical scientists. But beware of its promises!
ChatGPT-type AI models are hideously energy-intensive both in the model-training stage and after their large-scale roll-out. And updating them is a whole scientific issue. More importantly, while we place a great deal of hope in AI for Good, it unfortunately carries huge potential for wrongdoers as well.
What do you mean by that? In your opinion, what are the main issues and challenges of our era surrounding artificial intelligence?
Michèle Sebag — It’s both terrifying and exhilarating. For example, we’ve been working for nearly 5 years with France Travail and ENSAE Paris on the use of learning algorithms to highlight employment offers that are likely to interest job seekers. Technically speaking, it’s the same principle as using data for algorithms that make recommendations on major retail websites. But from an ethical viewpoint, it’s quite different. On Netflix, the same film can be recommended to a very wide audience, but France Travail cannot recommend the same person to a whole host of recruiting firms.
Then there’s the issue of bias. Men and women do not share the same criteria. For example, it has been observed that women are more likely to accept a lower salary if it means working closer to home. This is what the data tells us, but do we want our recommendations to encourage this, for example by showing women positions that are less well paid but nearer to where they live? How do we avoid setting such biases in stone within the models? And vice-versa: it isn’t our role to tell people what’s good for them.
can you tell us about your career and how your Inria project team came about?
Marc Schoenauer — We met at the École Normale Supérieure (ENS) in Paris. I began my career in 1980 with CNRS at the Applied Mathematics Centre of the Ecole Polytechnique. Michèle chose to start with a post in industry, before joining the École Polytechnique for her PhD in Solid Mechanics. So we were able to form a small cross-disciplinary team to work on optimization and learning. In 2001, I joined Inria in Rocquencourt. In its beginnings, the Inria Futur Centre (later to become the Saclay Inria Centre) enabled us to team up with LRI, the joint laboratory of Université Paris-Sud and CNRS, to create our TAO project team.
Michèle Sebag — TAO stood for “Thèmes, apprentissage et optimisation” (Topics, learning and optimization). At the time, learning and optimization made an original combination. Nowadays, they clearly go hand in hand. That meant we could get a multidisciplinary team together and we had a lot of fun. For example, we worked on the game Go, so it felt as if we spent our whole time playing! In 2016, following the authorised 12-year existence granted to the Inria project teams, TAO became TAU (TAckling the Underspecified, the aim being to highlight the absence of specification and the lack of awareness of what could go wrong in the field of AI.
What is your project team working on now?
Marc Schoenauer — Learning remains our core business, understanding learning algorithms and enhancing them, in terms of energy consumption, for example. (This is known as frugal learning). But we are also convinced that we have to widen our scope of expertise in order to enhance creativity and face up to the competition, even without the same material resources. Since the team was created in 2003, it has seen a wide variety of researchers. Today, for example, it has a core of three researchers in statistical physics, as we thought their expertise could be very useful to AI since they’re used to handling large volumes of data. We’ve also had researchers in “pure” mathematics, bioinformatics and even sociology. At the time, it was quite unusual to see such a diversity of expertise in a computer science team. Times have changed and we now welcome all fields of expertise.
Michèle Sebag — Among the team’s fundamentals is the fact that, considering the scale of AI uses in day-to-day life,
It is very important that algorithms do not become black boxes. In other words, we must create models that do not merely make suggestions, but also explain the reasons behind their recommendations. This means the reasoning has to be determined — which brings us back to the question of bias and the need to identify and correct it.
Another important aspect is the necessity to create causal models that are not based solely on correlations. Between the use of an umbrella and the fact that it’s raining, for example, a correlation can be established but not a causality; if everybody uses an umbrella, that won’t make it rain. This is a vital point because people think that AI and machine learning will allow us to predict, and therefore control, what will happen. But influencing reality goes beyond the usual remit of machine learning; such intervention requires causal models which are more powerful and considerably more difficult to build.
Finally, there’s the whole field of AI for the common good. Some of our colleagues work with the Environmental Ecology Laboratory; there’s farmland in Saclay where certain farmers use pesticides to eliminate parasitic insects and hence increase their yield. Unfortunately, those pesticides also destroy pollinating insects and therefore reduce the rate of pollination. We need to be able to qualify and quantify, with the help of AI, the service rendered to farmers by pollen-gathering insects.
Marc Schoenauer — Another interesting research orientation is the coupling of AI and digital simulation. The aim is to create calculation methods based on actual data rather than mathematical models, in order to speed up simulations and even incorporate phenomena that equations cannot accommodate. Indeed, with the support of Inria Startup Studio, three of our former PhD researchers are now launching a startup – AUGUR – devoted to this topic!
What can we wish you for the future?
Together, we intend to continue doing research, not just for the benefit of science, society and the environment, but also because we enjoy it. I’d like to point out that a key factor in efficient research is the atmosphere that reigns within the team. As long as it’s fun, everything goes well!
In that case, we wish you lots of fun!