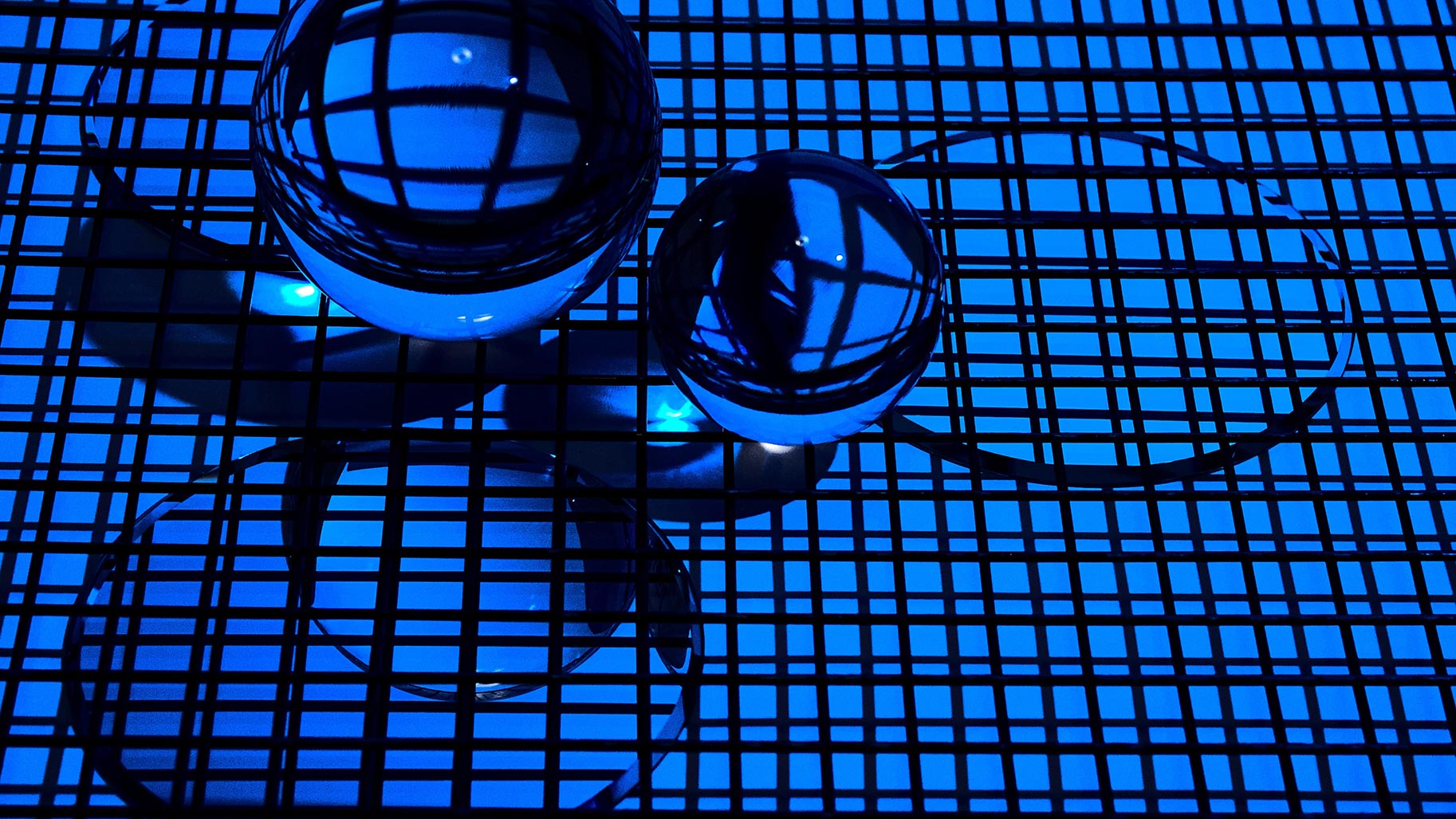
Fairness and respect of privacy, two key concerns
Among the various fields of AI, machine learning consists in training a model to respond to a problem by introducing a multitude of examples representing the task to be accomplished. But what happens when this model proves less effective for one group of people than another? It is no longer fair. ‘Let’s imagine a medical application where the goal is to detect suspicious moles’, Michaël Perrot, researcher with the Magnet team, begins. ‘Data changes according to skin tone, and that can raise problems of fairness. If certain moles are identified less effectively in a group, these patients won’t go to see a dermatologist and risk developing a serious illness which could have been avoided.’ This type of bias could notably emerge when the vast volumes of data on which these models are trained are not representative of the population as a whole.
In the medical example above, the training data can be collected from hospital consultations. However, each hospital has only a partial view of the problem via its local population. It will be difficult therefore to obtain a fair model. One solution consists in using decentralised learning to cross-reference data sources and thus enrich them.
How does it work? Several entities communicate together, with a cooperative aim and without sharing potentially sensitive data or storing this information in a single location managed by a third party. Data sovereignty is an important factor here, yet it does not guarantee an adequate level of privacy for the individuals present in the learning databases. ‘Even if the data is not exposed directly, the models it trains can be used to find sensitive information’, Michaël Perrot explains. ‘We thus need to develop specific learning mechanisms which make this impossible.’
Developing more fair models
To address this issue, Michaël Perrot launched a project entitled ‘Fairness in privacy preserving decentralized learning’, backed by the STaRS Grant which supports scientific research talents based in the Hauts-de-France (Northern France) region.
The aim of this project is to design new learning algorithms that respect privacy and learn models that do not discriminate against certain groups of individuals, in a decentralised manner, he tells us.
The first step of the project is to develop an algorithm to learn fair models that is as simple as possible, to which additional restrictions can be added, i.e. privacy and decentralisation. ‘We thus created an open-source method called FairGrad (Fairness Aware Gradient Descent)’, says Michaël Perrot. ‘It enables training of fair models in a simple way and is compatible with “Pytorch”, one of the standard machine learning libraries. It’s based on the principle of example reweighting. The idea is to increase the importance of disadvantaged individuals while reducing the impact of advantaged subjects. Our current objective is to combine this FairGrad method with the DecLearn software for decentralised learning, developed by our Magnet team.’
Studying the interplay between fairness, privacy and decentralised learning
In addition to algorithmic development, one specificity of the project is to explore the issue from a theoretical perspective to answer several questions that arise when imposing fairness constraints. How does fairness interact with decentralised learning? How does it interfere with privacy preserving machine learning? And lastly, how do the three concepts interact together? While the fields of fairness, privacy and decentralised learning have been widely explored individually, their interactions have received less attention in the current scientific literature.
Michaël Perrot thus looked into the impact on fairness of privacy constraints. With his co-authors, he demonstrated that this impact is in fact very limited when the data set used to train the model is sufficiently large. ‘The variations in terms of fairness are Bounded by the distance between the model respecting privacy and the model which doesn’t’, he explains. ‘The closer they are, the smaller the variations, and the impact of privacy constraints on fairness is more limited. There are several methods to train private models close to non-private models for sufficiently large data sets. Intuitively, the larger the subject population, the harder it becomes to identify one individual with certainty, which is commonly known as blending into the crowd.’
Human-centred AI, for the good of all
Beyond this particular research initiative, the question of AI and fairness is also a matter of concern for international institutions. Europe recently took up the subject. The Artificial Intelligence Act (AI Act), the European Commission’s AI regulation project, mentions fundamental rights in terms of data protection, human dignity and non-discrimination. ‘This is why fundamental research in AI is crucial’, Michaël Perrot concludes. ‘It enables us to better understand why biases can emerge in learning models, to study ways to avoid them and, above all, progress further in the field of human-centred AI. ‘It enables us to better understand why biases can emerge in learning models, to study ways to avoid them and, above all, progress further in the field of human-centred AI.
Find out more
General audiences:
- Le Parlement européen ouvre la voie à la régulation de l’IA [The EU Parliament opens the way for AI regulation], Le Point, 14/6/2023.
- L’Agence des droits fondamentaux de l’UE met en garde contre les algorithmes biaisés [EU fundamental rights agency warns against biased algorithms], Euractiv, 9/12/2022.
- Intelligence artificielle : la Défenseure des droits appelle à replacer le principe de non-discrimination au cœur du projet de règlement de la Commission européenne [AI: The Defender of Rights calls for the inclusion of the non-discrimination principle in the EU Commission’s regulation project], 21/6/2022.
- Magnet prescribes federated learning to healthcare , INRIA, 9/5/2022.
- Les discriminations algorithmiques | 2 minutes d'IA [Algorithmic discriminations | 2 minutes of AI] (video), Sorbonne University, 10/11/2021.
Experts:
- Differential Privacy has Bounded Impact on Fairness in Classification, by Paul Mangold, Michaël Perrot, Aurélien Bellet, Marc Tommasi, ICML, 2023.
- FairGrad: Fairness Aware Gradient Descent, by Gaurav Mahesharwari, Michaël Perrot, Transactions on Machine Learning Research, 8/2023. FairGrad software.