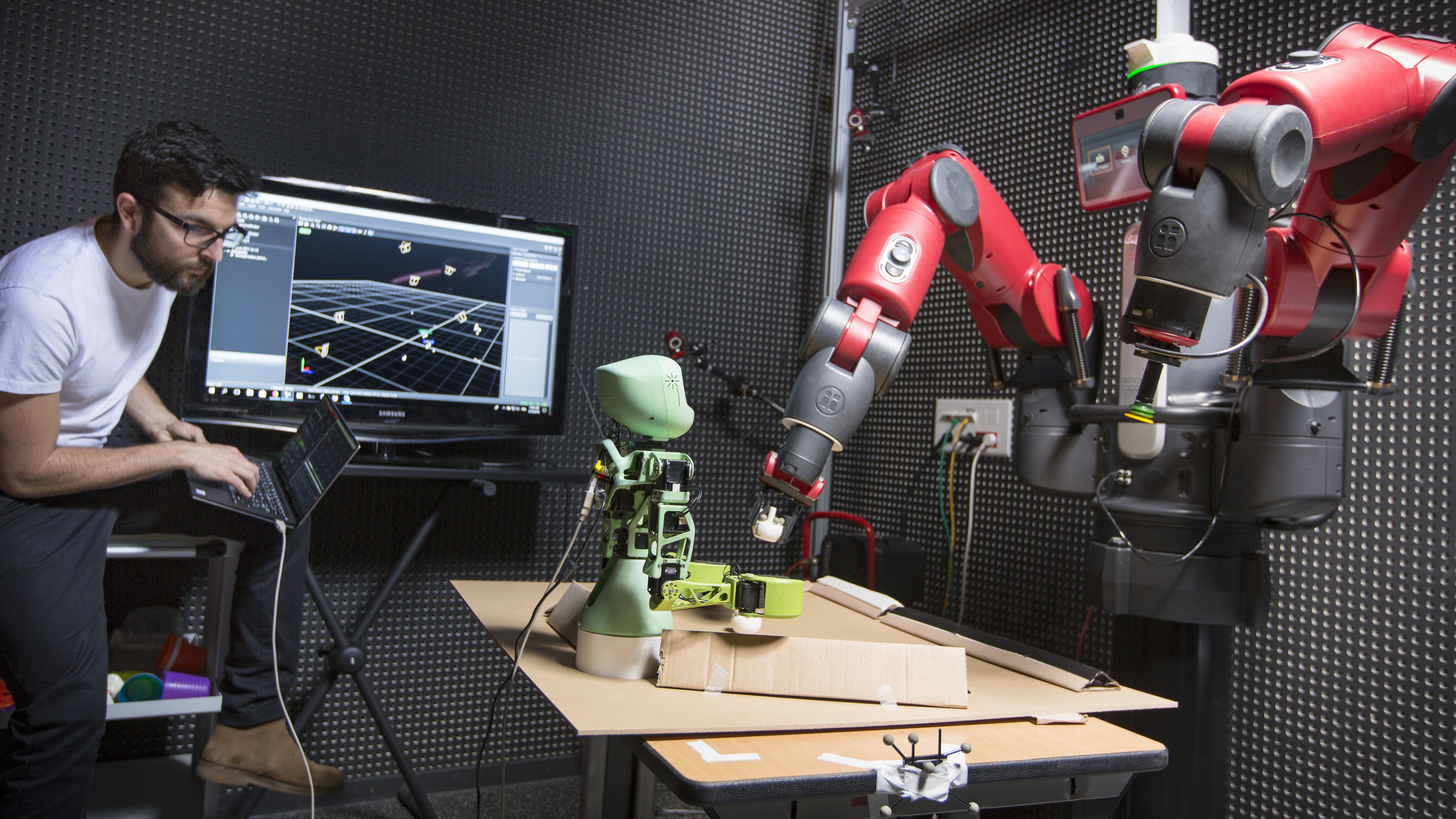
Flowers and Mnemosyne: at the heart of AI
Flowers: the project team pioneering “artificial curiosity”, an approach to artificial intelligence involving intrinsically motivated autonomous learning, where the goal is to develop educational technology capable of supporting human learning.
Mnemosyne: the project team developing models of neural networks. They mimic cerebral decision-making mechanisms in an open and changing world, corresponding to the executive functions to be deployed in order to detect change and adapt to it; this has resulted in the team considering different cognitive mechanisms, such as emotional evaluation of situations, simple decision making and its automation, motivated decision making, planning, reasoning, attention and working memory. They work alongside neurobiologists in order to decipher these circuits and to build adaptive neural models, the goal being to develop a better understanding of the brain and cognition, with a particular focus on medical applications (Parkinson’s disease, OCD, addiction, Alzheimer’s, etc.). Their work also has repercussions on the fields of machine learning (through the development of new bio-inspired algorithms) and artificial intelligence (cognitively plausible decision making models), with transferral over to the business world (industrial applications involving decision making, planning, the temporal organisation of behaviour, language processing, robotics, autonomous behaviour, education science, etc.).
CQFD and RealOpt: data science and optimisation
CQFD: the project team developing expertise in the optimisation of random and dynamic systems. The scientific issues tackled by the team cover both supervised and unsupervised learning, reinforcement learning, stochastic algorithms, genetic programming and evolutionary algorithms (in a theoretical, methodological and applicative context) and are applied to the fields of health (automatic tuning of cochlear implants, classification of EGG signals) and defence (stochastic filtering, adaptive control, etc.).
RealOpt: the project team tackling problems relating to combinatorial optimisation. This involves selecting a solution to a complex problem from a wide range of options as quickly as possible. Many artificial intelligence related problems can be formulated as optimisation problems, including clustering problems. The techniques developed by RealOpt have been used to solve complex strategic planning problems facing SMEs and major organisations such as the SNCF or EDF.
Geostat and Potioc: images and signals, human-machine interface
Geostat: the project team developing analysis methods for complex natural signals, using analogies and models taken from statistical physics. These models provide summaries of critical phenomena (including phase changing, for example) encountered while analysing large quantities of data. The team carry out research into the classification and analysis of signals in earth science and in health; we are working on a new class of predictive models and estimates based on data
Potioc: the project team exploring and designing artificial intelligence tools for analysing and classifying brain signals(particularly electroencephalographic (EEG) signals). This is taking place within the framework of their research into brain-computer interfaces, enabling users to interact with computers entirely through their brain activity. They design and develop artificial learning tools in order to denoise, characterise and classify EEG signals in a way that is both reliable and adaptive, in addition to using and developing AI tools designed to model users interacting with systems, focusing on their status and their preferences. The goal here is to provide interaction that is both adapted and adaptive, in order to maximise the efficiency and the effectiveness of the interaction. This technology is targeted at applications such as motor rehabilitation (after strokes, for example) or cognitive rehabilitation (e.g. improving alertness or attention) through BCI (Brain Computer Interface), or educational systems tailored to meet the needs of users.
Auctus: robotics
Auctus: the team focused on human-robot cooperation in the context of industrial applications. For effective cooperation, it is necessary to be able to control a robot’s capabilities in terms of autonomy and interaction through four aspects of equal importance: the first relating to its capacity to analyse its environment using sensors, including vision or pressure sensors, for example; the second relating to fine sensorimotor coordination, requiring command of control laws; a third relating to analysing and interpreting human activity, particularly with regard to gestures; and a fourth relating to the form the human-robot cooperation will take, including problems regarding the sharing of representations, the sharing of authority and, in a more general sense, decision support, not to mention issues surrounding acceptability and ethics.
Tadaam, Hiepacs and Storm: Specific optimisation, material components and architecture
Hiepacs: the project team focused on the optimisation of sequencing strategiesfor deep learning frameworks such as TensorFlow or PyTorch, the goal being to simultaneously boost parallelism and to limit memory consumption. The team work to develop efficient parallel algorithms using linear and tensor algebra for clusters of hybrid machines (CPU+GPU), algorithms which are then applied in the context of classification for metabarcoding.
Storm: the project team developing tools and methods for high performance computing in order to enable more effective use of parallel machines. Storm pay particular attention to difficulties with expression in parallelism, to difficulties relating to the implementation and the sequencing of calculations and communication and to difficulties regarding execution on heterogeneous system architecture, blending CPU, GPU and accelerators.
Tadaam: another project team developing tools and methods for high performance computing in order to enable more effective use of parallel machines. The tools developed can be used for the optimisation of inputs and outputs (storing and reading large masses of data for learning) and communications involving these masses of data in supercomputers.
Carmen, Monc, Pléiade and Sistm: biology and health
Carmen: the concept behind this project team is to improve the way in which heart rhythm disorders linked to the heart’s electrical system are understood and treated by developing effective and precise simulation models and tools and by using the available electrical signals in order to improve diagnostics. In addition to standard modelling and simulation tools, Carmen use and develop AI tools in order to design reduced models, the objective being to restrict the space of the parameters and to carry out simulations in real-time (e.g. using an endocardial catheter during an ablation, or simulating the propagation of electrical waves), to solve inverse problems for the reconstruction and interpretation of electrical signals - with neural networks or optimisation techniques, for example - and, in a more general sense, to incorporate experimental or medical data into our models.
Monc: the project team developing artificial intelligence algorithms in the context of their research into cancer, assisting with diagnosis in order to improve disease monitoring. The purpose of this is to better customise cancer progression models based on patient populations and the processing of medical data. The team also pay close attention to how reliable these methods are.
Pléiade: the project team developing algorithms for shape recognition and machine learning.Their goal is to discover patterns in the genetic diversity of organisms and to use these in order to build networks of interdependent functions and interacting organisms. Pléiade derive cellular and ecological process models, building software frameworks for applications in ecology, evolution and biotechnology.
Sistm: the project team developing and using applied statistical learning methods in health, primarily in clinical immunology and vaccinology. The application objectives for this team are to accelerate the development of vaccines, to understand and predict their effects and to optimise their administration, employing strategies from both supervised and unsupervised statistical learning for the analysis of high-dimensional data (multiomics) taken from groups of participants in clinical trials or observational cohorts. Individual optimisations and predictions make use of dynamic population models (ODE). The team also evaluate predictions and strategies developed among the wider population (clinical trials).
Cardamom and Memphis: Modelling
Cardamom: for this project team, who specialise in designing effective and reliable digital methods for simulating fluid flow with complex fronts, learning is the most important step when it comes to building metamodels and simplified modelscapable of reliably reproducing response surfaces during the process of varying physical parameters.
Memphis: for this project team, who have set themselves the target of deploying new digital models on high-performance computing infrastructure and making them available in order to meet real-life industrial needs, the convergence between modelling and data represents something of a new horizon in the context of digital simulation. This new strategy can be broken down into two components: learning the space solution to a physical problem, and designing ad hoc closing models combining distributed measurement systems and non-linear regression strategies.