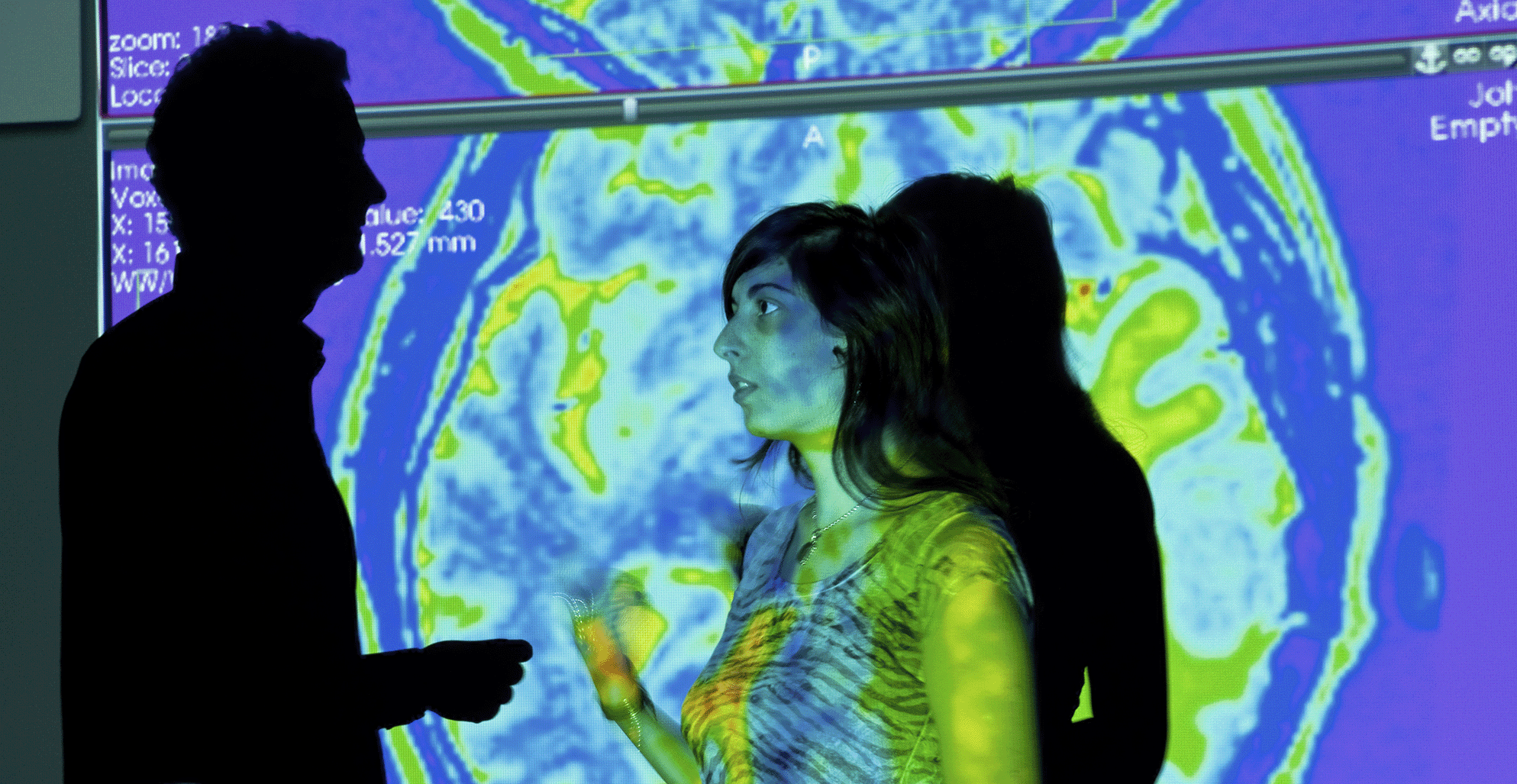
Machine learning is a branch of Artificial Intelligence (AI). In a nutshell, it involves feeding software thousands of examples so that it learns to carry out identification tasks, e.g. looking through images to identify dogs or cats. Beauty spots or malignant melanomas. In theory, this should open up a wide range of applications in medicine. For example, x-rays are collected from thousands of patients suffering from the same condition - what is known as a cohort. Then, using this machine learning data, the computer will detect the same visual characteristics in any new images taken during screening for other individuals. This becomes the target data.
In its April 2022 issue, the scientific journal npj Digital Medicine published a paper highlighting the gap between scientific investment and actual clinical progress made in the field. The paper was authored by Veronika Cheplygina from the IT University of Copenhagen and Gaël Varoquaux, a director of research at the Inria Saclay centre.
From an IT perspective, research efforts are primarily focused on improving the performance of algorithms, the aim being to make them more discriminating, and to ensure that they do in fact detect areas of interest. This race to see who can produce the best model has prompted a frenzy of scientific papers, while also leading to the emergence of what can best be thought of as a permanent league table organised by Kaggle, a platform within the Google galaxy. But in practice, from a medical perspective, this research activity “has had little clinical impact”, much to the chagrin of Gaël Varoquaux, head of the Soda [1] team at the Inria Saclay centre and co-author of a study [2] breaking down the mechanisms at play behind this paradox.
Biases that distort the model
A number of different phenomena are working together. Firstly, there is the issue of there not being enough data to train the algorithm. “When a data set is too small, it’s easy to get a visible performance with it, but this does not indicate a broader performance. The problem here is that, in medicine, there are few large cohorts. Those we do have access to are too small in relation to the complexity of the methods we employ and the complexity of the problems to be solved. 1,000 people isn't enough. Even 10,000 might not be. 100,000 would be enough for us to start seeing things. But obviously that’s difficult to get your hands on, particularly for rare diseases.”
What’s more, this training data sometimes contains biases, which can then distort the model. “In dermatology, some algorithms have been trained on images where malignant carcinomas had been circled in pencil by doctors. These algorithms didn’t look beyond the carcinomas that had already been circled. The same thing happened with pneumothorax, only this time it was a chest drain that was messing up the learning.” When there was no drain on the target data, the algorithm didn't detect anything.
Several aspects to rethink
The paper also noted that research into machine learning wasn’t necessarily focused on clinical fields where this technology could have the most impact. “If you look at competitions between algorithms, you find a lot dealing with lung x-rays. But as far as we are aware there is only one dealing with mammograms, despite the fact that there is most to be gained in detecting breast cancer early, when treatment is at its most effective. The chances of survival are extremely high. Looking at it logically, from a medical perspective, machine learning for this type of cancer should be prioritised.”
As far as improvements to the algorithms themselves are concerned, these tend to be negligible. “Scientists are putting more and more effort in, but the gains in terms of performance are increasingly small.” The authors scrutinised eight competitions organised by Kaggle: for lung cancer, prostate cancer, schizophrenia, intracranial haemorrhage, etc. In five of the eight, the gain for the algorithm finishing first was so low that it was below the margin of error capable of having an impact on the measurement.
Another peculiarity is what is known as overfitting. This is when a statistical model is extremely finely-tuned so that it completely matches one particular data set. Doing so boosts performance for this precise data set, but the algorithm will then suffer from a lack of efficacy when dealing with other data. “There comes a point when you need to stop fiddling about with things.”
Reading between the lines, there is also an issue with research sociology. “Our incentive systems are not fit for purpose. Scientists are ranked based on the number of papers they have published. It's as though they were being ranked by the mile and encouraged to write more lines. IT specialists continue to improve their algorithms on the margins. They publish a lot. But there comes a point when they stop working on the actual problem and it doesn't serve any purpose. They then have to approach it from a medical perspective. But that’s not easy for someone with a background in mathematics. This is a case of two truths colliding. One deals in numbers, axioms and formal problems. The other deals with a patient’s circumstances and their condition.”
How can this gap be bridged? By creating interdisciplinary communities? “Experience tells us this is what we need to do. That said, it’s not enough on its own. IT specialists have to get out of their comfort zones. They have to go and speak with the doctors using their algorithms. That might be tough, but it’s essential. One of the aims of our article was to put an end to this cognitive dissonance, forcing people to come to terms with the problem.”
Interdisciplinarity as a reinforcement
So how do we bridge the gap between these two worlds? By creating interdisciplinary communities? "Experience proves that we need to do this. That said, it is necessary but not sufficient. Above all, the computer scientist must get out of his comfort zone. He has to go talk to the physician who is the user of his algorithms. This may hurt, but it is essential. One of the goals of our article is precisely to break the cognitive dissonance that exists to force people to realize the problem."
[1] Soda is a research team in machine learning applied to health and social sciences (epidemiology, prevention...).
[2] Machine learning for medical imaging: methodological failures and recommendations for the future, by Gaël Varoquaux, Veronika Cheplygina (Inria, McGill University of Montreal, Mila Montreal and IT University of Copenhagen), npj Digital Medicine, April 2022.