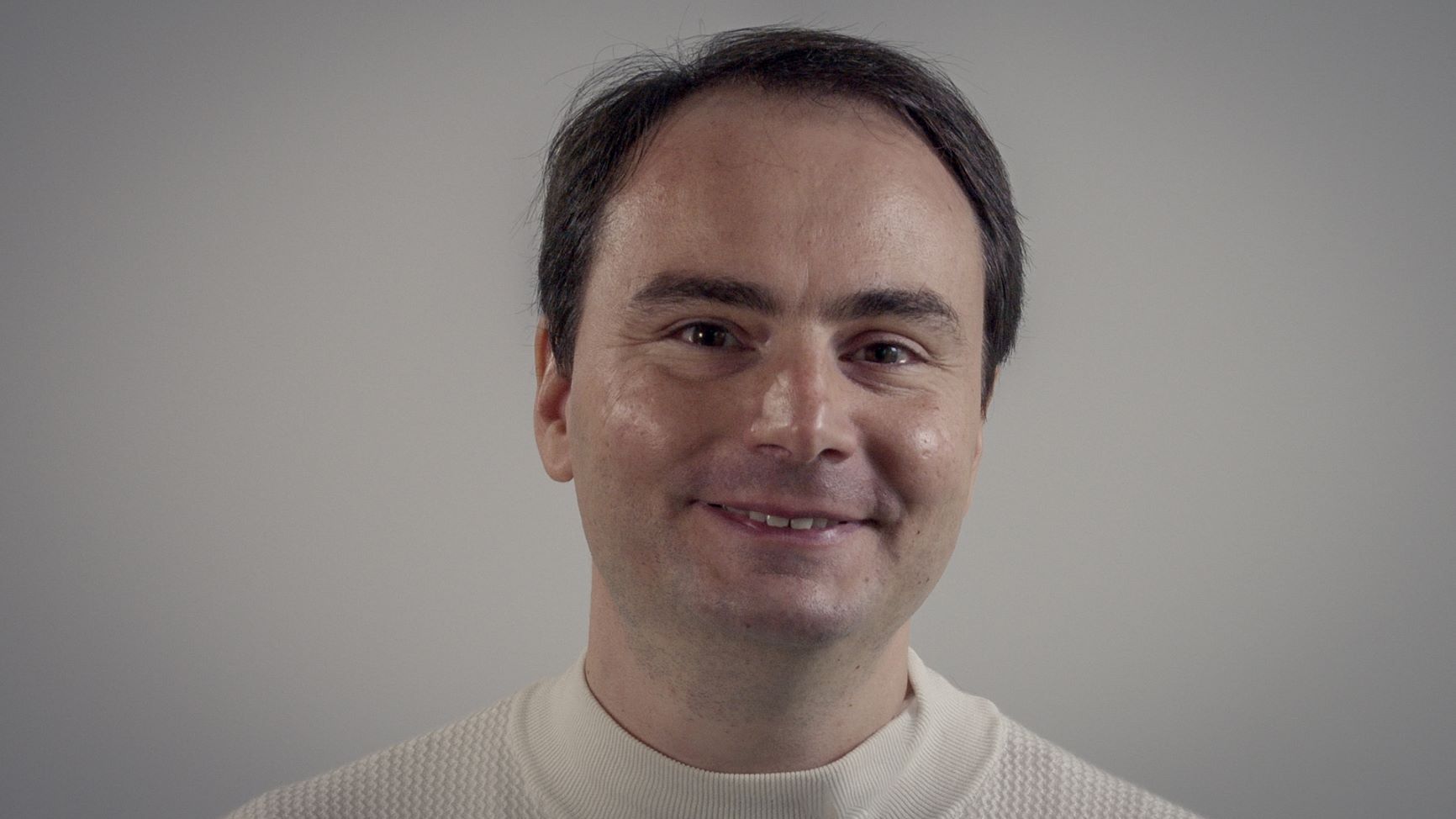
Anticipating through modelling
What Stanley Durrleman enjoys about mathematics is its capacity “to model the world around us, helping us to develop a deeper understanding of the phenomena found within it.” He also enjoys the way in which this language can be used to anticipate what happens and to get a grasp on a system.
There were many people who influenced Stanley Durrleman along his path towards becoming a researcher. While it was Nicholas Ayache who guided him towards digital medicine, it was through Alain Trouvé, then a researcher at the Mathematics Centre at the ENS in Cachan and joint supervisor for his PhD, that he understood the power of mathematical modelling, particularly when used in conjunction with digital experimentation. The range of different people he worked with at the ICM also encouraged him to integrate new markers into his models (genetic and psychometric data, data from sensors, etc.) in order to better tackle the neurological challenges lying ahead of us.
In light of this, it wasn’t much of a surprise when he decided to study for a Master’s degree in applied mathematics in 2001. The computer science aspect would come later, when he understood how it could be used for digital experimentation purposes. Studying mathematics gave Durrleman first-hand experience of mathematical modelling, allowing him to test these models against reality using measured data.
More than life science in itself, what he found himself drawn to most were complex problems. He was less interested in the actual application - it could just as easily have been physics or engineering. As circumstances would have it, when he began his PhD in 2006, he joined Asclépios, a project team headed up by Nicholas Ayache, a pioneer in the computer analysis of medical images.
As a result, medicine - via medical imaging - was the field he found himself working in, with a particular focus on neuroscience. In terms of complexity, this gave him plenty to be getting on with.
Building a history of the brain
In his research, Stanley Durrleman draws on data from what are known as “longitudinal studies”: series of medical images and clinical observations from different stages in patients’ lives. Although everyone is different, once this individual data is aggregated, you can then build a general overview of the brain, its structure, how it works and how it develops over time.
But what are these models used for? Primarily, in a basic research context, “these digital constructions can be used to map changes to the brain during ageing”, explains the researcher, both in normal circumstances and in people with neurodegenerative diseases. By comparing different trajectories, it is then possible to come up with hypotheses regarding the different biological mechanisms at play.
Understanding the development of neurodegenerative diseases
These models are also a cause for optimism from a clinical perspective. In France alone, there are more than a million people suffering from neurodegenerative diseases. These conditions are often diagnosed late, when the first symptoms appear, but changes can be detected in the brains of patients anywhere from ten to twenty years prior to the disease manifesting itself. Owing to a lack of effective treatments, having access to a tool capable of identifying these warning signs would help in anticipating diseases and improving patient care.
What has made his life more difficult
Factoring in the dynamic aspect wasn’t straightforward. Machine learning works really well with something that is fixed, but it’s more complicated to predict what will happen to a patient over time, particularly when you're not seeing them that often. In the end, it was differential geometry which helped him to overcome this obstacle from a conceptual point of view.
But this isn’t just about diagnoses. These models will also enable prognoses to be made by building a picture the way in which these diseases progress over long periods of time, often decades. Modelling long-term changes to the brains of people with these conditions, using data from both structural and functional neuroimaging and data on brain metabolism, reveals the changes and dysfunctions that can take place within these processes. It also helps to develop an understanding of what causes different types of symptoms and, eventually, the diseases themselves.
Decision-support aids for treatment
Stanley Durrleman has been modelling the progression of brain conditions for a number of years now. In 2016, he was awarded a prestigious ERC Starting Grant to help him develop methods and algorithms for building these dynamic models. Five years down the line, these methods and algorithms have been proven to be highly reliable when it comes to predicting the evolution of clinical and radiological signs in patients as much as three to four years in advance.
What’s more, “these are soon to be implemented into a decision-support system used by neurologists at the Memory and Alzheimer’s Disease Institute (im2a) at Pitié-Salpêtrière hospital.” Durrleman has also expanded his research to cover Parkinson’s disease and Huntington’s disease, demonstrating the general nature of the algorithmic principles underlying the models he has developed.
Stanley Durrleman in five dates
2010: won second prize at the French Computer Society’s (SIF) Gilles Kahn PhD awards
2011: joined the Aramis project team (Inria and the Brain and Spine Institute - ICM)
2016: awarded an ERC Starting Grant for his research into the use of dynamic modelling for cerebral ageing
2019: became the first European winner of the Sanofi iDEA Awards
2020: awarded the Inria - French Academy of Sciences Young Researcher Prize
Better treatment through more targeted therapeutic trials
These models also meet an ambition of going further when it comes to therapeutic management. There are currently very few drugs capable of treating these conditions. Many trials have failed and those rare treatments that are available deal largely only with symptoms. We might be inclined to think that this is because the right drugs just haven’t been found yet. But Stanley Durrleman has another hypothesis: “These therapeutic trials also fail because they’re badly designed. They're based on the premise that the disease progresses at the same rate at all stages.” As Durrleman’s research has shown, however, the opposite is true. And because it is difficult to select patients at very specific stages, there is a significant risk of the desired effect being lost. The researcher believes that the tools he has developed will make it possible to identify patients at exactly the right stage of their disease in order to demonstrate the effectiveness of a treatment.
But how would this work? Some signs of brain atrophy and certain neurological evaluations constitute “signatures” that can be used to place individual patients on a certain trajectory with regard to a given illness. “This means we can select those who, in terms of the target function of the drug being tested, are immediately before the decline phase”, explains Durrleman. What’s more, during therapeutic trials, it will also be possible to check for any significant variations in the group who are given the candidate medication compared to the control group. Durrleman’s research attracted the attention of the pharmaceutical laboratory Sanofi, which presented him with an iDEA Award, in addition to a partnership contract in the context of developing a highly promising candidate drug for Parkinson’s disease. This gave the team access to additional expertise, in addition to giving them the opportunity to test their experimental model against concrete examples.